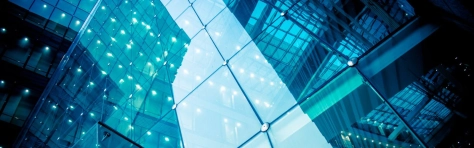
Reporting requirements for MiFID investment firms
This article explores the key aspects of the Internal Capital Adequacy Assessment Process (ICAAP) for MiFID investment firms, as outlined in the Investment Firm Regulation (IFR) (EU) 2019/2033.
In this article by Gary Stakem, director of actuarial and risk services, he outlines the implications for insurers and how actuarial teams can adapt to meet new needs.
With AI set to cause disruption right across the insurance value chain, compliance with the AI Act will become a key discussion point in boardrooms for years to come. The journey from understanding the Act to achieving actual compliance will present significant challenges, demanding a broad range of skills. These include experience with complex models, handling large datasets, a deep understanding of the commercial and regulatory environments, and effective stakeholder communication. While all of these competencies are already central to the actuarial profession, some additional upskilling will be necessary.
For decades, actuaries have utilised large volumes of data, written code and built statistical models for assessing risk, pricing, and product design. This role now looks set to evolve following the recent surge in data availability and computer processing capacity. Actuaries are now transitioning towards providing oversight for machine learning analysis, ensuring algorithms are explainable, ethical, and compliant with regulatory standards.
The Act, which defines AI in the broadest terms, seeks to categorise AI systems into unacceptable risk, high-risk, limited-risk, and minimal-risk.
Unacceptable or prohibited risk systems include those intended to manipulate or deceive and those which exploit vulnerabilities.
High-risk systems are permitted but will be highly regulated under the Act. The list of high-risk systems explicitly includes risk assessment and pricing of life and health insurance, along with evaluation of an individual’s creditworthiness. Although not explicitly called out, general insurance systems that profile individuals could also be considered high-risk.
The Act will require insurers to employ staff with a sufficient level of AI literacy. Actuaries, with their robust experience in complex models, are uniquely positioned to bridge this gap. The legislation offers actuaries an opportunity to upskill, broaden their influence, and play a critical role in ensuring the responsible use of AI.
Actuaries are ideally placed to act as compliance officers for high-risk systems under the AI Act.
Under the Act, insurers must establish, implement, and maintain risk management systems for high-risk AI models. Actuarial teams can facilitate requirements to conduct model testing against defined metrics and probabilistic thresholds, ensuring models are performing consistently for their intended purpose. Actuarial judgment will be crucial in mitigating residual risk in pricing and underwriting models to acceptable thresholds.
Moreover, the Act requires that training, validation and testing datasets adhere to appropriate data governance practices, a concept well-developed in actuarial modelling to date.
Monitoring and feedback loops are key features of any robust risk management system and high-risk AI systems are no exception to that. The Act specifically references models which continue to learn after deployment. Actuaries must address how to prevent their models from learning biases post-production.
Many actuaries working in the data science domain have already started developing underwriting risk assessment models by training neural networks (a specific type of machine learning model) on insurers’ own datasets. The recent rise of a specific type of neural network, Large Language Models (LLMs), has introduced a whole new world of capabilities. LLMs (which include ChatGPT) are designed to interact with human language and are trained on vast amounts of text data, including information available on the internet. Insurers are now on the cusp of combining their own internal data with the vast superpower of LLMs to develop risk prediction models of far greater sophistication than has ever been seen before.
While this technology is incredibly powerful, the models used are becoming increasingly complex and are subject to all the disinformation and biases on which they are trained. When it comes to risk rating, claims assessment, or any other insurance activities that directly impact consumer outcomes, it is critical that the models used are fair and unbiased. To ensure this is the case, models must be explainable. Given the nuances of AI applications in insurance, actuaries will play a pivotal role in explainable AI, or XAI.
Explainability is a cornerstone of the AI Act. Actuaries will face challenges in establishing XAI frameworks as traditional linear models give way to more complex non-linear ones. Overcoming these challenges is crucial to detect biases and ensure fairness in models.
In some limited circumstances, the AI Act will actually permit the processing of special category data (race, ethnic origin, religion, genetic data, political beliefs, etc.) in a test environment so biases can be detected and mitigated from live models. How this gets executed in practice will subject to much ethical debate.
Model explainability, though not new to actuaries, demands sharp governance practices. Key aspects of XAI include:
Compliance with the AI Act will be a top priority for insurers in the coming years and so actuaries with appropriate competencies will be much sought after.
Actuarial professionals looking to upskill should consider the following:
As the EU AI Act ushers in a new regulatory framework for insurers, actuaries are poised to play a central role. By leveraging their traditional skills and embracing new responsibilities, actuaries can become instrumental compliance officers overseeing the responsible use of AI.
This website uses cookies.
Some of these cookies are necessary, while others help us analyse our traffic, serve advertising and deliver customised experiences for you.
For more information on the cookies we use, please refer to our Privacy Policy.
This website cannot function properly without these cookies.
Analytical cookies help us enhance our website by collecting information on its usage.
We use marketing cookies to increase the relevancy of our advertising campaigns.